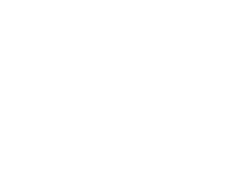
Blog
by Michael
Friday, July 18, 2025
Articles & insights of interest in public management & leadership for the week ending July 18, 2025
by Dan Chenok
Tuesday, July 15, 2025
How OPM's strategic digital modernization can securely reduce processing times, boost calculation accuracy, and improve the overall experience for annuitants and their survivors.
Thursday, July 10, 2025
In this essay, I present ten insights from my conversation with Denise Linn Riedl, highlighting key strategies and themes for leveraging technology to enhance municipal service delivery and resident engagement.
by Dan Chenok
Tuesday, July 8, 2025
Agentic AI is rapidly reshaping the way organizations operate, promising a new era of autonomy, adaptability, and mission impact. But what exactly is Agentic AI, and how can federal agencies harness its power to overcome longstanding challenges and deliver real results?
Thursday, July 3, 2025
In this essay, I reflect on the key themes and insights from my conversation with Amanda, exploring how technology informs governance and can shape a better future for local governments and communities.
by Dan Chenok
Wednesday, July 2, 2025
Earlier this year, the IBM Center for The Business of Government and the National Academy of Public Administration brought together a broad group of experts and stakeholders for a roundtable discussion.
Friday, June 27, 2025
Articles & insights of interest in public management & leadership for the week ending June 27, 2025
Wednesday, June 25, 2025
I’ll unpack the key insights from my dialogue with Faisal Hoque, distill actionable leadership lessons, and provide practical recommendations for government executives to navigate this AI-driven era responsibly and effectively.
by Dan Chenok
Monday, June 23, 2025
In today's rapidly changing world, governments must adapt and evolve to meet the ever-growing needs and expectations of their citizens.
Friday, June 20, 2025
Articles & insights of interest in public management & leadership for the week ending June 20, 2025