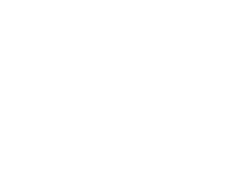
How Can Government Manage Risks Associated with Artificial Intelligence?
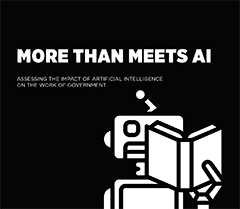
Co-Author: Tatiana Sokolova, IBM
With contributions from Claude Yusti and Anna Lenhart with IBM, and Peter Kamoscai with the Partnership for Public Service
___________________________________________________________________________________________
Greater access to and sharing of data can help increase the understanding of how best to address underlying risks and ethical issues in AI implementation.
Over the past 18 months, the IBM Center for The Business of Government and the Partnership for Public Service have collaborated to research how artificial intelligence (AI) can help government agencies deliver positive outcomes for their constituents, drawing on practical experiences and lessons learned first addressed in an earlier report on AI with the Partnership.
To gain insights on this challenge, the Center and Partnership co-hosted a series of four roundtables that explored pressing issues surrounding AI in government, discussed effective practices for addressing these issues, and developed actionable recommendations. Each session was conducted in a non-attribution setting to promote candid dialogue among participants. The first roundtable, held in July 2018, focused on potential uses for AI. The second roundtable, held in October 2018, focused on AI and its workforce implications. Drawing from the insights of these roundtables, the Center and the Partnership released our first special report that addressed how government can best harness AI’s potential to transform public sector operations, services, and skill sets. The third roundtable occurred this past February and addressed how data, culture, and technology influence the policy decisions that government agencies need to make.
To conclude this series, the fourth and final roundtable was held on May 14. The session brought experts together for a discussion on how government can best address the ethical concerns and other risks, including bias when delivering AI solutions. Below is a summary of the key questions and findings from that discussion.
Key questions:
Risk Management:
- What are the most significant risks associated with the use of AI in government?
- While AI systems mature, how should government address risk?
- How might agencies transition from a culture of risk avoidance to one of risk tolerance?
Ethics:
- What ethical concerns should agencies anticipate when using AI? How can agencies address these concerns?
- What principles or guidelines should agencies follow to address issues of value alignment, bias, data quality, cybersecurity and privacy when working with AI?
- What key issues drive data quality in AI systems? How can agencies develop approaches to recognize and respond to data that may encode bias or misrepresent the population?
Summary of Key Findings from the Roundtable
Current Challenges
Governments will see an AI transformation occur over the next 15 to 30 years. However, agencies face several challenges in implementing AI technologies:
- Lack of training and skills
- Lack of a comprehensive AI strategy
- Lack of engagement with academic experts
- Gaps in existing policies in government, particularly around risk classification
- Access to data, specifically a lack of inter-agency data sharing
- Barriers to procurement
Current Initiatives
The Government of Canada
The Government of Canada has taken a “tools, rules, people” approach to ensuring responsible AI implementation by civilian agencies. They have drafted a Digital Playbook to assist agencies in becoming more agile, open and user-focused. Canada also set up an agile procurement vehicle that asked vendors to demonstrate proficiency in AI ethics. The vehicle was designed to promote an iterative process, and to promote increased digital innovation and transparency.
In addition, to help assess and mitigate risk, Canada developed an Algorithmic Impact Assessment. The tool rates proposed projects from 1 to 4, with 1 as low impact level and 4 as high. These ratings are all available online to foster transparency, and each rating level is associated with a different set of agency. Finally, the Canadian School of Public Service started a Digital Academy pilot to upskill current employees, all of whom moved on to more meaningful work -- saving 19,000 hours with no job loss.
Research from the Administrative Conference of the US (ACUS)
ACUS, in collaboration with Stanford and NYU Law Schools, is currently mapping use cases of machine learning and AI to objectives for improved adjudication and enforcement in Federal regulatory programs. This initiative is intended to help agencies look through program data and classify risks to improve ex ante regulatory decisions. This would involve greater focus on how to use real world data. The full report on this project will be available later on this year.
Both of these examples demonstrate that addressing ethical concerns within AI will require a multi-disciplinary approach that brings people with different expertise (such as engineers and lawyers) to the same tables.
Areas of Opportunity
While some government initiatives aim to tackle challenges like those discussed above, the following issues need further focus to address risk and ethical imperatives in order to realize the opportunity that AI technology brings:
- The Need for Explainable Algorithms
- Applying Ethics within a Cost-Benefit Framework
- Data Governance
- A Strategic Vision for AI Use
The Need for Explainable Algorithms
Machine Learning (ML) algorithms are only as good as the data provided for training. Users of these systems can take data quality for granted and can come to over-trust the algorithm’s predictions. Additionally, some ML models such as deep neural networks are difficult to interpret, making it hard to understand how a decision was made (often referred to as “black box” decision). Another issue arises when low quality data (i.e., data that embeds bias or stereotypes or simply does not represent the population) is used in un-interpretable models, making it harder to detect bias. On the other hand, well-designed, explainable models can increase accuracy in government service delivery, such as a neural network that could correct an initial decision to deny someone benefits for which they are entitled.
Research into interpretability of neural networks and other kinds of models will help build trust in AI. More broadly, educating stakeholders about AI – including policy makers, educators, and even the general public -- would increase digital literacy and provide significant benefits. While universities are moving forward with AI education, government needs greater understanding of how data can impact AI performance. Government, industry, and academia can work together in explaining how sound data and models can both inform the ethical use of AI.
Applying Ethics within a Cost-Benefit Framework
One roundtable participant observed that AI ethics is to AI policy as political philosophy is to law or regulation. In other words, AI ethics is less a problem to “solve” than a set of norms and frameworks that inform decisions. Therefore, AI ethics should be applied in specific contexts (e.g., for what reasons and for who is AI used?) and levels of understanding (e.g., how much AI is appropriate for a given scenario?).
One way to apply ethics involves the practice within policy making of cost-benefit analysis. Such methodologies would allow agencies to compare the risks associated with AI (e.g., potential for human harm, discrimination, funds lost) with the benefits (e.g., lives saved, egalitarian treatment, funds saved) throughout the lifecycle of an algorithm’s development and operation. Cost-benefit analyses often include scenario planning and confidence intervals, which could work well in evaluating AI systems over time—provided that the “costs” considered include not only quantifiable financial costs, but more intangible, value-based risks as well (such as avoiding bias or privacy harms). Done correctly, this approach could provide a clear way to communicate risk and decisions about how and when to use AI -- including risks of leveraging AI to support a decision, relative to risks of decisions based solely on human analysis – in a way that informs public understanding and dialogue.Data Governance
Data Governance
To ensure confidence in data used by AI and other automated decision systems, proper data governance including testing and audits will be necessary. This testing could focus on data quality, security and data user rights, and ensure that automated decision systems are not discriminatory. Another element of data governance could set-up protocols for inter-agency data sharing, which can increase efficiency but also introduce privacy risk -- privacy protection within AI has engendered divergent views, and using a risk management perspective allows for agencies to assess how much personal data they need to collect and store based on the benefits to the data subjects.
Finally, governance protocols can help clarify how and when to acquire data, especially from outside parties. Government’s use of third-party data to support the use of AI for regulatory decisions may differ from that used for research or analysis. And there may be inherent vulnerabilities in third party data that call for mitigation strategies in a risk management framework.
A Strategic Vision for AI Use
Government employees often lack familiarity with AI technology, and are understandably skeptical about its impact on their work. Greater engagement across agencies in setting forth needs and priorities, defining factors that can promote trust in AI systems, and developing pathways to explain the technology, could enhance understanding of AI’s impact across the public sector. Sharing best practices is especially important given the differential levels of maturity in AI use across agencies and even levels of government. With greater understanding of and involvement in the technology, agencies can promote understanding of the benefits and risks associated with AI and foster a cultural shift in supporting responsible AI adoption.
Conclusion
Roundtable participants noted the need for more education, understanding, and skills involving AI; better data to inform AI systems; and clearer guidelines for responsible AI implementation. At the same time, a perfect AI system, one free of all ethical constraints, will never be actualized. The more practical path forward in understanding and addressing underlying risk is to engage early with developing AI prototypes, iterate on the solutions, and learn from the results. Some participants felt that the risk of not using AI may be greater than any risk from responsible and ethically designed systems. Risk-based approaches that include public dialogue provide a starting point.
In the end, AI is about data. Agencies can build greater understanding of the benefits and risks of AI, and help employees and citizens within an ethical framework, by reaching decisions that emerge from responsible use of the right data.
***
For additional information about issues concerning AI risk management, ethics and bias in the public sector, this video provides an extensive discussion (video overview, background information, and panel discussion) of the subject at a recent panel from the American Society of Public Administration's Annual Meeting, organized by Asim Zia of the University of Vermont.
Read the Nextgov article, "Can Government Manage Risks Associated with Artificial Intelligence?"
Read the ExecutiveBiz article, "IBM’s Dan Chenok: Data Governance, Explainable Algorithms Could Help Agencies Address AI-Related Risks."