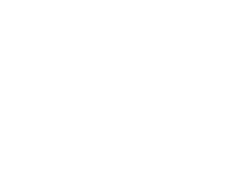
Digital Transformation of Serious Games in Federal Sector
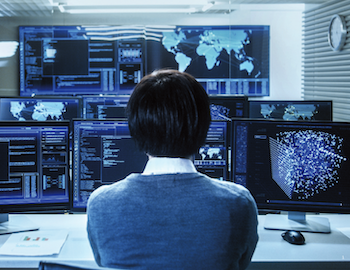
Guest Blog Authors: Phaedra Boinodiris, Trust in AI Business Transformation Leader, IBM Consulting and Stephen Gordon, Strategic Accounts Director, Defense & National Security, Red Hat.
The current workforce has grown up with technology and is comfortable adapting to new devices and functionality, so it makes sense that serious games have become a popular tool. In recent years, serious games have become a topic of interest for defense organizations to assist in training, planning, and improving employee engagements. In this post, we discuss serious games and applications in the federal sector.
1 – Introduction
Serious gaming is a form of advanced simulation but it differs from traditional simulation in that player decisions could guide the progression of a simulation. Artificial Intelligence (AI)/Machine Learning(ML)/Decision Optimization could assist players to make more effective decisions, providing recommendations and/or real-time impact assessments of choices.
2 – Evolution of Serious Games
In the federal sector, defense organizations have been in the forefront of applying serious games and advanced simulations. Over the last decade or so, defense programs have been hard at work curating their data and process models to be ingested by advanced simulation systems so that organizations can simulate a wider variety of different scenarios in collaborative multiplayer environments. Organizations such as these cannot rest on their laurels assuming that the hard work is done, for it has just begun.
US and allied defense leadership agree that in order to meet the demands of the rapidly evolving future operating environment, the need to develop courses of action for progressively complex dilemmas is paramount to plan for conflict, avoid surprise by unforeseen crises, and fully grasp the implications of shifting global challenges. The information and cyber domains have become particularly challenging, serving regularly as a live battle lab for adversaries testing techniques and tactics, state sponsored operations, attacks on smart power grids, autonomous vehicles, the Internet of Things, and the growing threats to deployed forces and civilian populations from disinformation weaponization. Modern technology platforms provide the ability to visualize information campaigns, similar to how military campaigns are viewed in the air, sea, cyber, and land domains, to better understand how to posture against competing campaigns simultaneously in all domains. Specifically, ML, natural language processing, intelligent analytical services -- technologies widely used in commercial “AAA” gaming engines – can be enabled on a unified, extensible technology platform. This can render 4K realistic terrain, progression of game narrative in individual campaigns, matchmaking, autonomous red-team challengers predicting every move, communications and team collaboration, even back-end services for live gaming operations, identity management, data processing, and video streaming services. Such an approach shows great promise in simulating future plans for military leaders who need to practice decision making against a thinking enemy under conditions of uncertainty.
The future of defense will include a race to information processing and decision-making, and the U.S. government could establish a sustained advantage by harnessing AI and autonomous systems. Speed is a major tenant of US defense modernization efforts. AI and autonomous systems are being employed in a growing number of military and commercial applications to aid and speed up decision-making to expand the reach and endurance of human operators.
In an increasingly complex multidomain warfare (MDO) environment, irregular warfare, information operations, psychological, and electronic warfare, etc., modern command and control designed for decision-centric operations and time, creates multiple dilemmas that disrupt an adversary's decision cycle such that the enemy would become more vulnerable to one to counteract another. Integrating human command with machine control further speeds the development of courses of action (COA's) and decision-making, is responsive across a whole range of scenarios, and puts command and control (C2) back in the hands of the warfighter. A move toward interoperable open data standards will help speed through volumes of data, assessing combinations of tactics and strategy.
The ability to process data in large quantities using AI models - operating in between gaps of contested cyber and electromagnetic environments, will give US forces a strategic advantage. The changing nature of war and conflict surface insights for what will be needed to shape a joint all domain command and control (JADC2) model. For instance -interoperability between disparate systems, data, transport systems, etc. will require a great deal of retrofitting, and significant investments in fielding AI to fuse data. This is critical as the ability to process and distribute information faster, relative to your enemy improves probability of success exponentially.
DOD’s recent efforts to implement AI and autonomous systems have mostly focused on improving current ways of operating, and could improve by developing new hybrid warfighting concepts, simulations for special operations, grey-zone factors, proxy warfare models, etc. As the data sources feeding simulations grow, and scenarios become more complex, the need for a trust "index" for data services, a source reliability and information credibility ranking - similar to how the A1-F6 rating index for intelligence source and information reliability is used for human data collection - will have to be applied in almost real time to artificial intelligence enabled sourcing. For example, confirmed information from a reliable source has a rating of A1, unknown-validity information from a new source without reputation is rated F6, an inconsistent illogical information from a known liar is E5, confirmed information from a moderately doubtful source is C1. Reasoning through massive amounts of data and determining the most reliable insights derived from disparate data sets using trustworthy proof, is a technical design challenge that holds tremendous promise for simulating complex multidomain problems and testing hypothesis and COA’s.
There is a co-evolution underway in the future design of the internet - web3 and blockchain for example - that will enable the next generation of powerful applications running on a cloud-enabled infrastructure. The better and more robust the infrastructure, the bigger the design space will be for application design. Proof systems, validity proofs, or “acceptable routes” for instance could be valuable in wargaming, saving a lot of time by proving a problem is solved without having to verify each problem has a solution - mathematically it is proven reliable. This may have potentially positive implications for instance in the adjudication process where significant time spent replaying and analyzing decision processes for instructional purposes or to understand success or failure of a strategic plan is reduced as there is an assumed, reliable trust in the decision process.
Trustworthiness and reliability in military decision analysis from an algorithm relies on reinforcement learning of clear motivations - training AI models to stay resilient, spread risk, and operate heterogeneously. The role of autonomy in this networked warfare is a difficult problem to solve. Communications will be interfered by the adversary -there will be gaps in signals from drones to ground to networks for instance, and exploit those gaps is where AI can assist. DARPA's Mosaic warfare expands on decision-centric operations by leveraging artificial intelligence and autonomous systems. Open data and communication will create pathways that can be readily published, subscribed by users and diverse data services, analyzed with relevant AI algorithms that reside at the tactical edge where time and precision is critical to mission success.
3 – Trust Considerations
As serious games evolve to tackle more and more complex systems, being able to ensure that people trust the results of the model becomes the priority. Without ensuring trust, organizations cannot extract value from their investments. To earn trust, organizations must resolve challenges with investments on three fronts. First, they need to nurture a culture that adopts and scales data and AI safely. Second, they need to create investigative tools to see inside black box algorithms. And third, they need to make sure their strategy includes strong data governance principles.
There are four major challenges that government agencies are facing when it comes to the implementation of AI:
- Government agencies often have an incomplete understanding of their data assets and do not have adequate data governance processes in place.
- It sometimes is difficult for agency officials to determine out how to assess risk or build emerging technologies into their missions. They are not certain whether to develop products in-house or rely on proprietary or open-source software from the commercial market.
- Operational tools must be human-centered and fit the agency mission. Algorithms that do not align with how government officials function are likely to fail and not achieve their objectives.
- AI and data management skills are in short supply. Oftentimes, AI knowledge is siloed.
Trustworthy data and AI depend on more than just the responsible design, development, and use of the technology. It also depends on having the right organizational operating structures and culture. To ensure fair and transparent AI, organizations must pull together task forces of stakeholders from different backgrounds and disciplines to design their approach. This method will reduce the likelihood of underlying prejudice in the data that’s used to create AI algorithms that could result in discrimination and other social consequences.
Task force members should include experts and leaders from various domains who can understand, anticipate, and mitigate relevant issues as necessary. They must have the resources to develop, test, and quickly scale AI technology. Having people trained to ask the hard questions about data are key including: What is the data lineage and provenance? Was this gathered with consent? Is it representative? Social scientists are trained to ask these hard questions and are incredibly valuable teammates.
Organizations should seek out tools to monitor transparency, fairness, explainability, privacy, and robustness of their AI models. These tools can point teams to problem areas so that they can take corrective action (such as introducing fairness criteria in the model training and then verifying the model output).
One oftentimes finds that in government agencies there is a misalignment between investments being made in AI and the agency’s overarching strategy. In fact we found that about 70% of investments in AI flounder in proof of concept. Design thinking frameworks help align misaligned siloes between the agency leaders and the technologists who understand the maturity of the data uses to power the models.
Organizations that incorporate design thinking practices in this way can also use them to help guide practitioners on how to discern unintended effects, pre-process data input and post-process data output to ensure that a model’s risk is being managed holistically. For example, it takes a significant amount of effort to render an AI model explainable to the layperson- taking the time to empathize about an end user’s experience is key.
Any organization deploying real data and AI in a serious game or simulation must have clear data governance in effect. This includes building a governance structure (committees and charters, roles and responsibilities) as well as creating policies and procedures on data and model management. With respect to humans and automated governance, organizations should adopt frameworks for healthy dialog that help craft data policy. More and more organizations are also using synthetically generated data in order to test AI models and run analytics on algorithms. This is the ultimate way to defend people’s privacy and stress test the accuracy of algorithms.
Gartner estimates that by 2030, synthetic data will completely overshadow real data in AI models.
Rolling out a data and AI governance program is an opportunity to promote data and AI literacy in an organization. Kicking off an AI governance effort with specialized synchronous classes can not only help educate stakeholders but galvanize them too so that they can be advocates for the new rollout. Finding a tech partner that can train your data science leaders to understand how to assess for the risk of their own models and to mitigate for that risk holistically is critical.
For the US government- obviously a very highly regulated industry- organizations should find specialized tech partners that can also ensure that the model risk management framework meets supervisory standards and that these standards are adopted by procurement offices such that they know exactly what kinds of audits, and features/function they need to see in AI models in order to meet standards.
There is no magic pill to making your organization a truly responsible steward of data and artificial intelligence. Earning trust in AI-powered serious games (and certainly AI in general) is a socio-technological challenge that necessitates a holistic approach.